Equipment Downtime Analysis with IoT Applications
Internet of Things (IoT), what can you use it for? Does IoT applications analysis with equipment downtime. These are frequently asked questions and it is not entirely unjustified. A relatively new concept requires text and explanation for understandable IoT examples and applications that are currently being put into practice within various sectors. No less than 42 percent of unplanned downtime in a factory is the result of machine failure. It is therefore not surprising that 71 percent of manufacturers who adopted an IoT project were initially looking for ways to reduce machine downtime. The main successes achieved in the execution of these projects are identified in the areas of efficiency (less downtime), lowering operational costs, increasing productivity, and understanding how equipment can be optimized. Empowered automation provides the best solution for IoT downtime in Chicago.
Why do we use IoT Analytics and its real applications?
It is an area of data science where data from sensors and connected electromechanical systems is analyzed and turned into valuable business insights. Industrial-grade IoT applications are called IoT (Industrial Internet of Things). Let’s discuss the industrial applications of IoT analytics.
What can you do with IoT in practice?
We can do smart monitoring, perform maintenance predicted for you, perform predictive maintenance, innovating your products and/or services, optimizing internal processes, making use of new revenue models, and much more.
IoT Applications occur in every sector.
Every sector has several applications related to the IoT (Internet of Things) Things. Even in the sector, you are in, we come across forms of IoT.
Below we will list several IoT examples that are currently taking place in daily practice.
Think of sectors such as:
- Maritime World
- Healthcare
- Security and Demotics
- Infrastructural Projects
- Industrial World
Trends in IoT Analytics:
With the rise of .com and the rise of connected devices, the use of IoT analytics is also increasing. Let’s take a look at Google’s global trends in IoT analytics from 2004 to 2019.
Typical IoT Analytics Flow:
A typical IoT analysis uses the following steps:
Data Collection:
A collection of data from IoT sources such as audio, image, light sensors. Processing streaming data is a major challenge for IoT applications.
Pre-Processing of Data:
Preprocessing collected data is a tricky part of machine learning use cases. Suppose that the function engineering for heart rate sensor data is very different from the data collected in weather stations.
Analyze Data:
Thorough exploratory data analysis is done in this step of the IoT analysis use case.
Train Under Test:
After preprocessing and EDA, different machine learning and deep learning models are trained per use case and business requirements. Business and technical KPIs are determined on a case-by-case basis. Based models are chosen from cross-validation and offline and online testing is performed.
Implementation and Prediction:
This is the part where systems respond to the insights gathered from the analytics solution. Based on the performance of the model, it is retrained or recalibrated.
You might also like
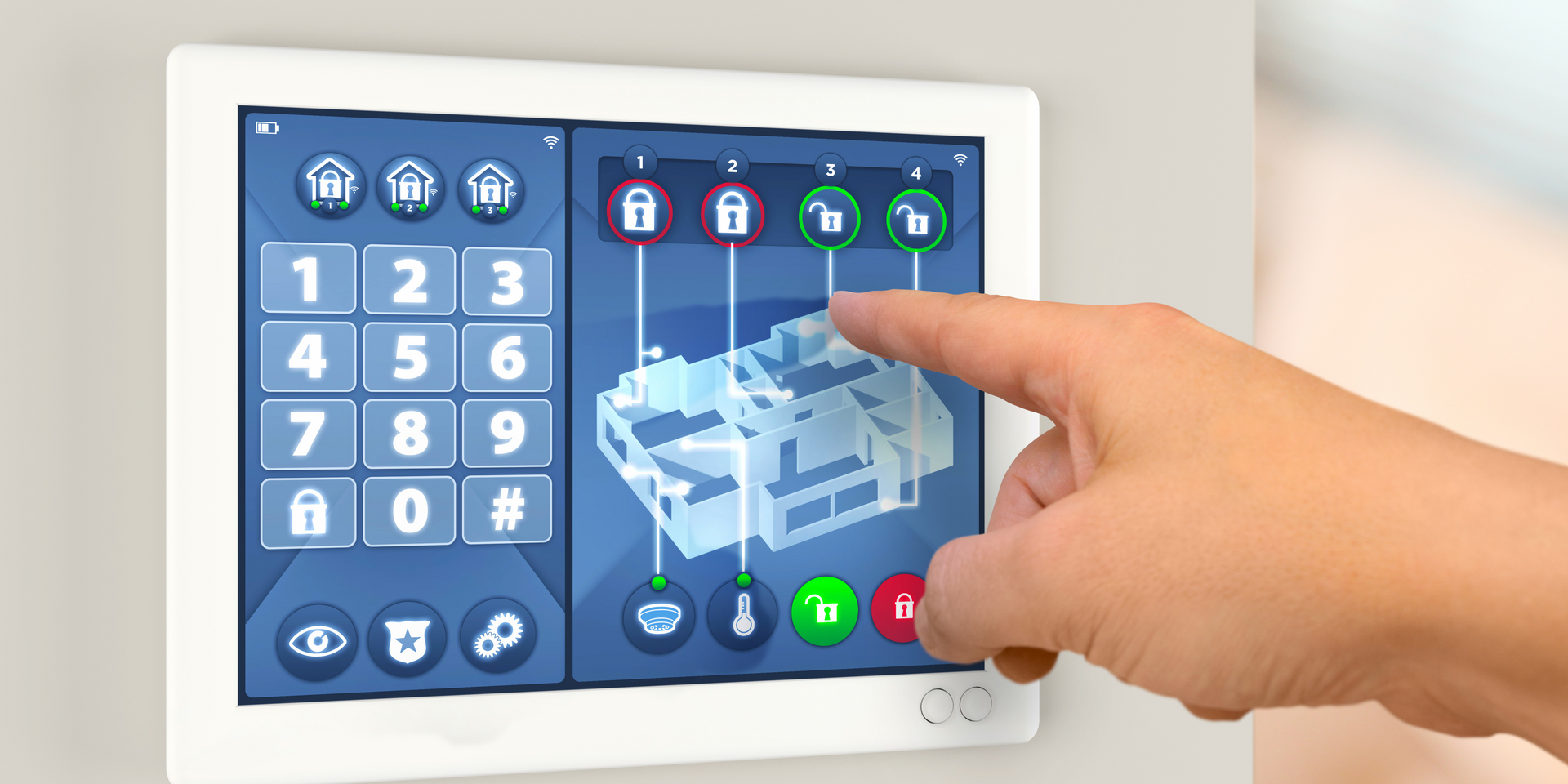
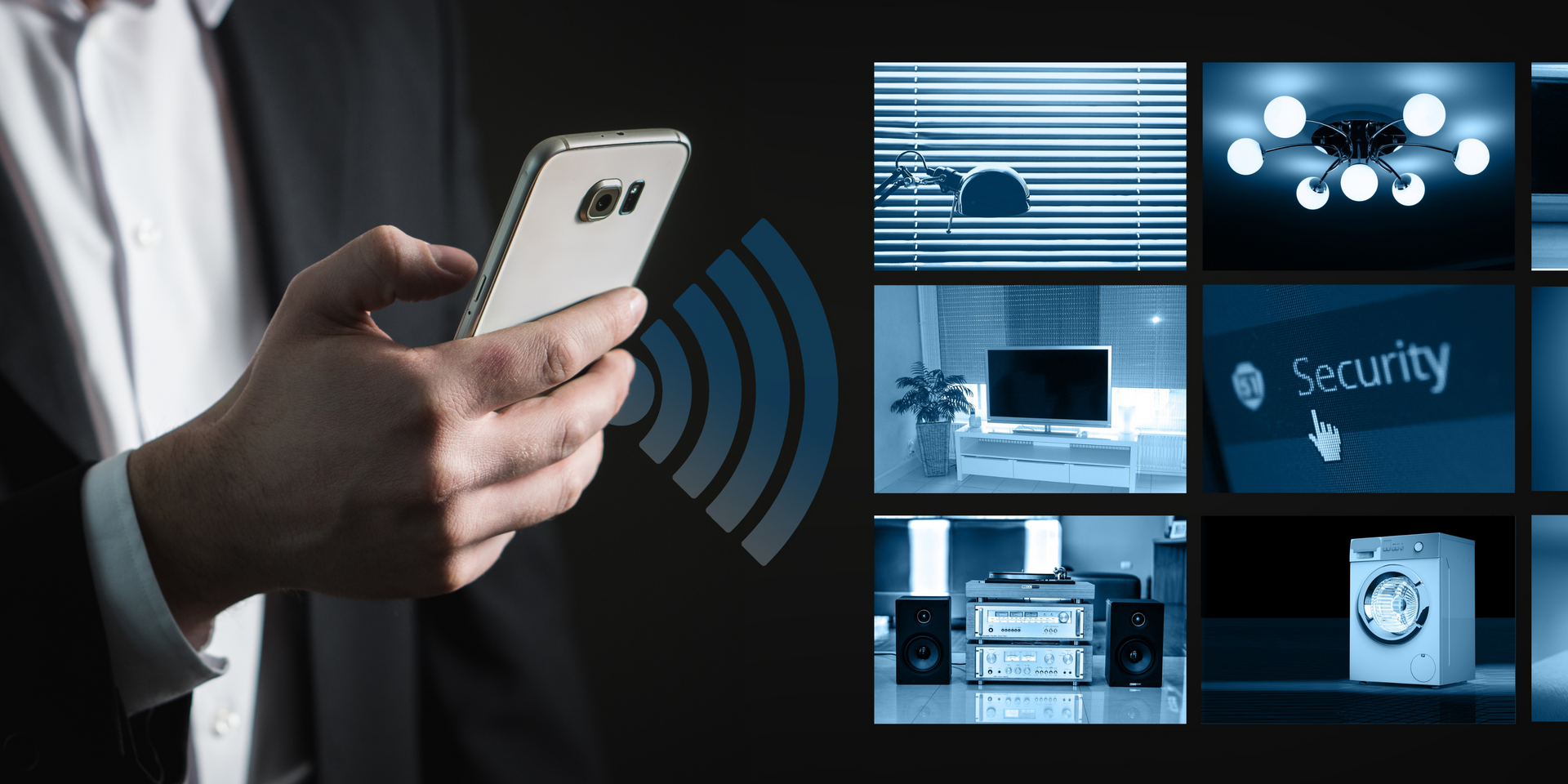
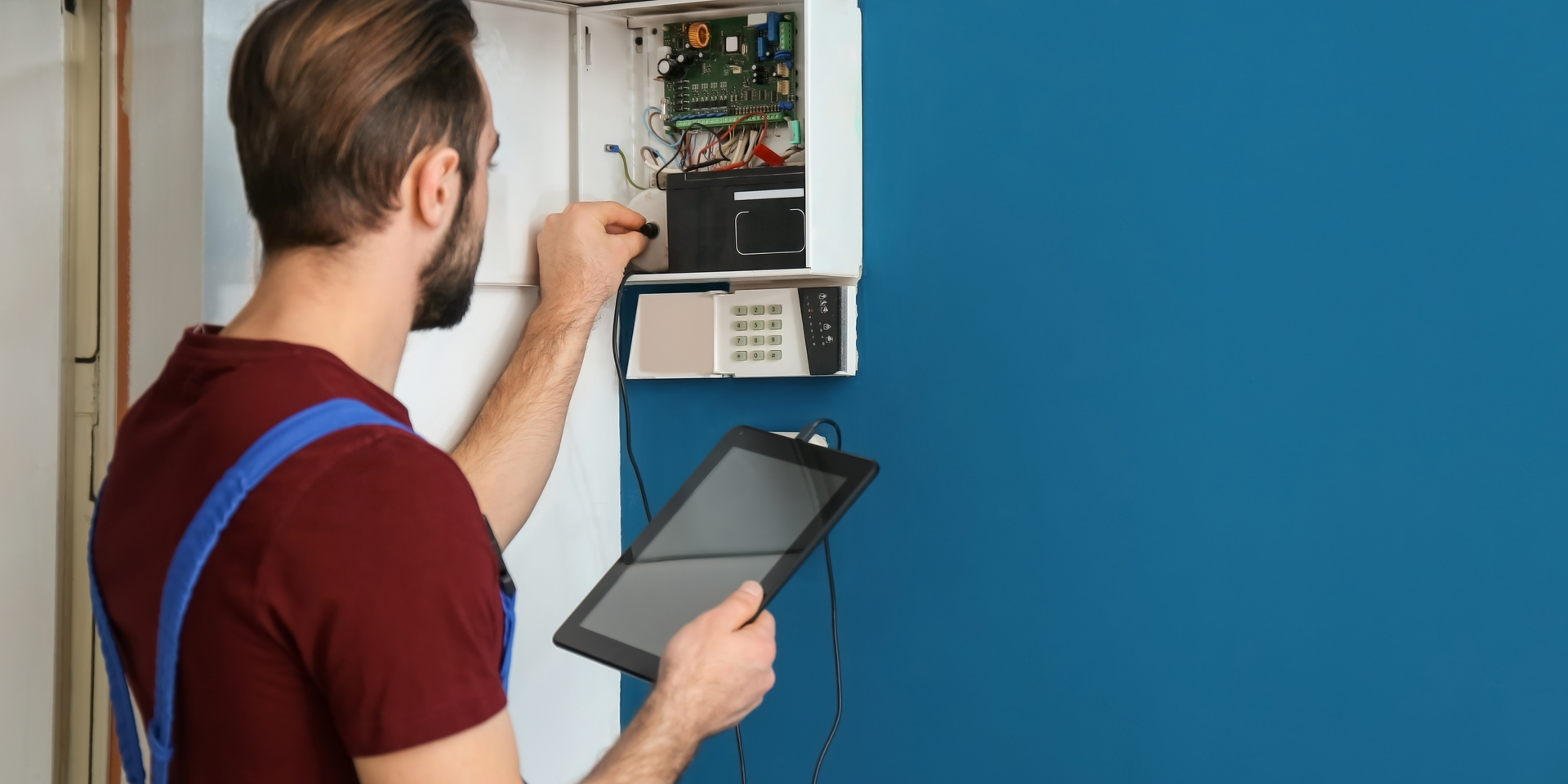